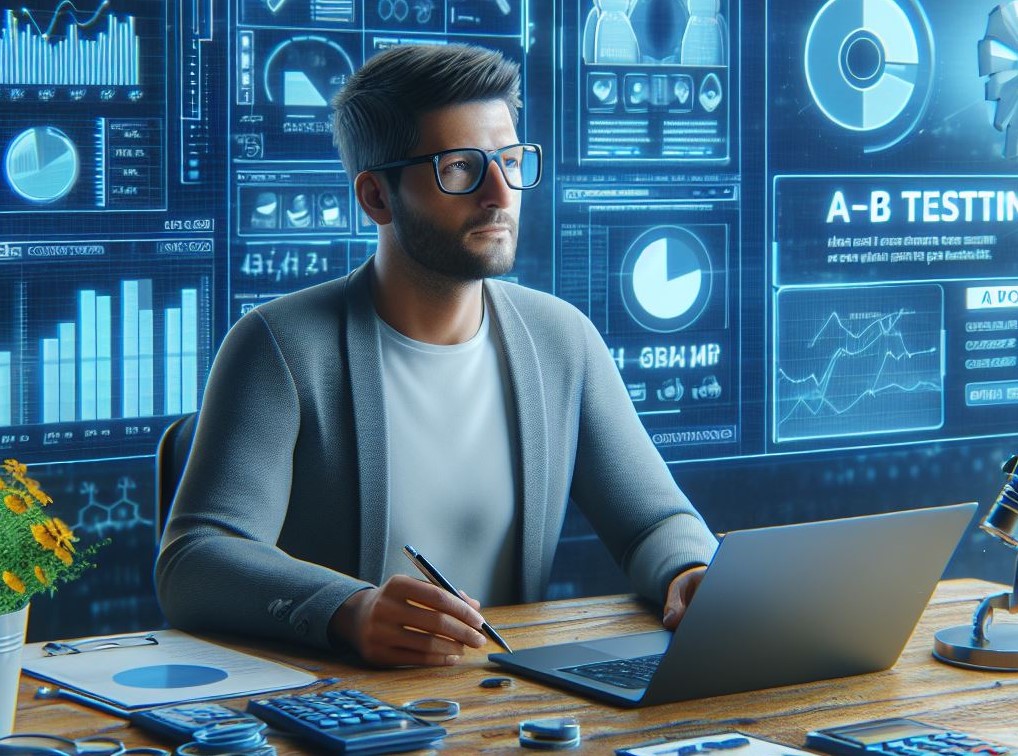
We often hear the mantra in paid advertising: “Always be testing.” But testing only pays off when it leads to better campaigns.
Personally, I believe in “always be testing… when you have a good hypothesis.” If you spot something that could be better and have an idea to improve it, go ahead and try it out. But don’t just throw random ideas and hope for the best. It’s crucial to approach A/B testing thoughtfully.
That way, when you see improvements, you understand why and can keep refining your strategies.
In this post, I’ll share some of my top PPC A/B testing examples and offer tips to help you conduct meaningful and impactful tests for your PPC campaigns.
Table Of Content:
A/B Testing Hypotheses
No matter which A/B testing example you choose to pursue, it’s crucial to stay focused on your hypothesis throughout the experiment. Here’s what I mean:
As mentioned earlier, always kick off your test with a clear hypothesis. What exactly are you testing and why? Avoid vague statements like “I think a new ad will perform better.” Instead, strive to articulate your intentions clearly.
From my experience, crafting a detailed hypothesis leads to more effective tests and produces actionable results.
For instance, consider this typical A/B testing example hypothesis: “I want to test automated bidding to see if it works better.”
While this is a valid test, what does “works better” really mean? A strong hypothesis typically includes specific details. Although we’ll delve into the specifics in the next steps, think of your hypothesis as a statement to your highest-ranking supervisor.
They may not grasp the intricate data you analyze daily, but they want a clear understanding of what you’re aiming to achieve.
Here’s a hypothesis to kick off your A/B testing: “Automated bidding will help us achieve lower CPAs on our main conversion action.”
To provide further guidance, here are some A/B test hypothesis examples for various experiments you might consider:
- “Utilizing cost comparisons in ad copy will distinguish us from competitors and improve ad performance.”
- “Expanding our market to a new state will increase market share without increasing overall advertising costs compared to our current geotargeted locations.”
- “Implementing a landing page with additional supporting content will enhance prospect engagement, resulting in a higher conversion rate.”
A/B Testing Strategies You Should Try
When it comes to testing hypotheses in platforms such as Google Ads and Microsoft Ads, there are various methods you can employ. Additionally, depending on the platform, you might find specific A/B testing tools at your disposal.
While there isn’t a definitive “wrong” way to test a hypothesis, each PPC A/B testing example has its own set of advantages and disadvantages to consider.
Sequential Testing (on/off method)
This first A/B testing method is often the simplest for advertisers to execute. You start by analyzing the data from your current setup, then make the necessary adjustments aligned with your hypothesis.
After running the campaign with these changes for a period, you compare the statistics to gauge the impact. It’s a straightforward process. For example, you might gather four weeks’ worth of data using your existing ad copy.
Then, you pause those variants and launch a campaign with cost-focused copy for another four weeks. Finally, you compare the performance metrics.
This approach has its advantages. It’s easy to implement and allows you to monitor the campaign for significant shifts in performance. However, there are some drawbacks. Since the variants don’t overlap, external factors like seasonal changes or budget constraints could affect the results.
Additionally, any other modifications to the campaign during the testing period could cloud the interpretation of the outcomes. Despite these limitations, sequential testing can still provide valuable insights.
Location Testing
In a geolocation A/B testing scenario, you maintain the existing campaign setup without any alterations. Instead, you create an experiment variant targeting a different location. This could involve expanding into a new market or targeting a subset of your current audience.
For instance, if your campaign covers the entire United States, you might test changes in specific states rather than nationwide.
To ensure the accuracy of your geolocation A/B test, it’s crucial to establish clear boundaries between your control and experiment groups. This involves setting up separate campaigns and excluding specific locations from your control campaign.
Unlike sequential testing, geolocation testing allows you to run both variants concurrently, facilitating a direct comparison of results. However, challenges arise from the inherent differences between regions.
Factors like regional preferences or market dynamics can influence campaign performance, making it challenging to attribute changes solely to the tested variables.
Split Testing
Split tests represent the gold standard of A/B testing, offering a solution to some of the limitations found in sequential and geolocation testing methods. However, true split testing is becoming increasingly challenging to execute due to advancements in platforms like Google Ads and Meta Ads.
These platforms utilize AI-powered machine learning to optimize ad performance, often favoring one ad variant over another based on campaign objectives or bid strategies. As a result, traditional A/B tests may not yield balanced results, as one variant may receive preferential treatment in the auction process.
To address this issue, platforms like Google Ads offer experiments and Facebook Ads provide split testing features. These tools allow advertisers to set up controlled tests focusing on single or multiple variables, ensuring each variant receives equal exposure and a fair opportunity to perform.
Analysing Performance in Various Testing Methods
Now that we’ve established how we’re going to conduct our tests, it’s crucial to define the specific PPC metrics we’ll use to gauge success. We can’t just settle for a vague notion of “performing better” – we need concrete criteria.
Firstly, we need to determine our primary Key Performance Indicator (KPI). Is it the cost per lead in Google Ads? Conversion rate? Click-through rate? Impression share? The choice will depend on our hypothesis and the A/B testing method we’re employing. Select the metric that most accurately reflects the success or failure of our test.
There are three common approaches to defining success metrics. Let’s say we’re aiming to improve the CPA (Cost Per Acquisition) for an account. Here are some ways I could frame my success metric:
- Target performance: This test succeeds if the experiment variable achieves a $60 CPA.
- Percentage improvement: This test succeeds if the experiment variable has a 10% lower CPA than the control.
- Statistical significance: This test succeeds if the experiment variable demonstrates an 80% confidence level of outperforming the control.
Each of these measurement approaches is valid. Choose the one that aligns best with your objectives and goals.
Definte Dealbreakers and Limits For Testing
Now, let’s delve into some additional metrics that are worth considering. While your primary focus might be on optimizing your cost per lead, it’s essential to recognize that other metrics may fluctuate as well. In fact, it’s highly likely that many of them will see significant changes. It’s crucial for you to determine what level of change in other statistics is acceptable.
For instance, you might be comfortable with a 20% decrease in click-through rate as long as the cost per lead reaches a profitable level. Alternatively, you might be okay with a slight increase in cost per click as long as revenue remains stable. However, not everyone is willing to tolerate significant fluctuations in other metrics.
Let me illustrate this with an A/B testing example involving various metrics: I once had a client who aimed to reduce the cost per lead on their branded terms by 20%, but they were adamant about maintaining an impression share of at least 80%.
While we knew achieving this balance would be challenging, we set up an A/B testing experiment for target CPA bidding to try and lower the CPA. However, as we delved into it, we realized that to meet our CPA target, Google only displayed the ads for about 60% of the potential impressions.
This discrepancy was a dealbreaker for the client, so we decided to halt the test and explore alternative strategies.
Think About Your Testing Schedule
Unfortunately, there comes a point when A/B tests must conclude, even if a clear winner hasn’t emerged. These tests can’t continue indefinitely; otherwise, you’ll never have the opportunity to test anything else.
However, it’s essential to strike a balance because A/B tests need to run long enough to gather sufficient data for decision making. While some very large accounts might consider making decisions after just one week of testing, in most cases, I recommend a minimum of two weeks and a maximum of two months.
Beyond this timeframe, tests become unwieldy, and other factors may start to invalidate the results.
So, regardless of the A/B testing example you choose to implement, ensure that within two months, you’ll have accumulated enough data to confidently determine whether your hypothesis was correct.
Summery
A/B testing is an essential tool that every marketer should consider incorporating into their ad accounts in some capacity. Before diving into any A/B testing example, it’s crucial to approach the test with a clear head.
This means having a well-defined hypothesis, a solid plan of action, and an awareness of potential deal breakers. By doing so, you set yourself up for success, regardless of the outcome of the test.