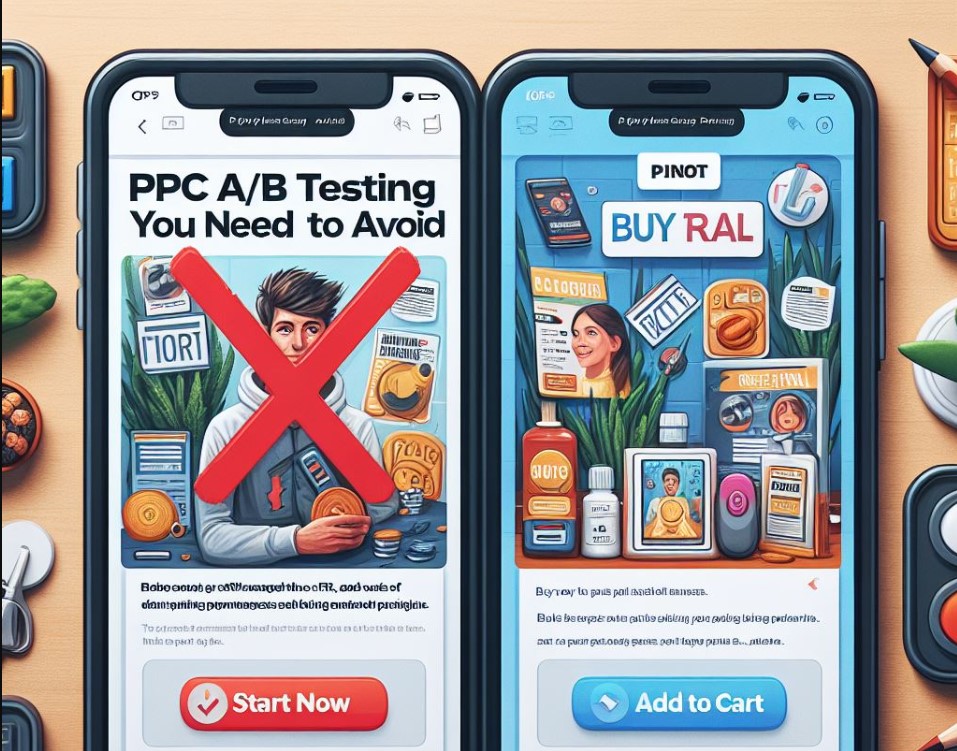
Have you ever tried out the best version of your ad after a split test and thought, “Hmm, why isn’t this working as expected?” It’s not just you, it happens way more often than we realize.
Split testing or A/B testing is supposed to be a game changer when you find your winner, but sometimes it misses the mark. There are traps you need to avoid, and that’s what we’re diving into in this article.
We’ll explore the common mistakes that mess up your PPC split tests and share some handy tips to make sure your tests actually give you the insights you’re looking for.
Don’t Aim For a 95% Significance
When you’re diving into A/B testing, you should start with a solid hypothesis. Something like, “If we add urgency to our ecommerce ad copy, we expect the CTR to go up by 4%” Nice and clear, right?
But here’s where things get tricky. Marketers often hear about reaching “statistical significance” for their results, and it can get confusing fast. If you’re not sure what that is, no worries you can learn about it here.
Now, in the world of PPC, some things are like magic spells that usually work, like adding urgency or showing limited stock. On the flip side, there are some tricks that might not do the trick, like throwing in complex lead forms.
Here’s the secret sauce: If you’re super confident (like 99% confident) that a quick change will bring in wins, just go for it. You don’t always need to do the whole A/B testing and statistical significance dance.
Now, you might wonder, “How do I convince my client that we can make this change without a full-blown test?” Here’s the game plan:
- Keep a record of your tests so you can show off success stories later.
- Check out what your competition is up to. If everyone’s doing the same thing, there might be a good reason.
- Share insights from articles with catchy titles like “Top 50 tests every marketer should know about.”
Your mission is to be a time-saving hero. And you know what they say – time saved is money earned.
Don’t Let Statistical Significance Stop You from Testing
Here’s the deal: Some marketers might say, “Only stop a test when it’s statistically significant.” Well, that’s sort of true, but not the whole truth!
Sure, hitting 95% statistical significance is a good thing. But here’s the catch – it doesn’t mean you can totally trust your results just yet.
When your A/B test tool says you’ve hit stat sig, it’s basically saying your control and experiment groups are different. Got it. But here’s the twist – it doesn’t tell you if your experiment group is actually better or worse than the control group.
So, reaching 95% stat sig doesn’t guarantee that your cell B (the experimental one) is consistently better than cell A (the control one). It could flip-flop, and that’s a headache.
Here’s the kicker: Your A/B test results become unreliable as soon as they hit 95% stat sig. How unreliable? About 26.1%. Oops!
Now, how can you make sure your results are the real MVP? First off, don’t rush to stop your tests just because they hit 95%. And second, tweak how you design your A/B tests. Let me break it down for you.
Evaluate Your Target Audience
Okay, let’s make this math talk simpler. Imagine flipping a coin. If you only do it 10 times, you might not be sure if the coin is perfectly balanced. Do it 100 times, and you get a better idea. Now, do it a million times, and you’re pretty darn sure.
The point? In PPC terms, it’s like this: A/B tests need lots of data to be reliable. If you’re dealing with a small audience (like 10 people), your test might not be as accurate. But with a bigger audience (1 million or more), you’re on the right track for trustworthy results.
Size Matters in Split Testing
ow, let’s talk about audience size in A/B testing. Not every project or client has a huge audience or lots of clicks. But here’s the deal: If you’re expecting small changes, you don’t need a massive audience. In fact, running tests that tell you what you already know is a waste of time.
So, what’s the sweet spot for a small change in results? Luckily, tools like A/B Tasty have a handy sample size calculator to help you figure it out. I’m not endorsing them, but their tool is user-friendly. You can also check out others like Optimizely, Adobe, and Evan Miller for comparison.
Here’s the trick: Use these tools and check your past data. Make sure your test can give you reliable results.
Segment Your Traffic Sources
Branded search traffic is gold compared to cold, non-retargeted Facebook Ads audiences. Picture this: Your branded search traffic spikes, thanks to a killer PR stunt, making your results look amazing. But, hold on, are those results accurate? Not really.
Here’s the scoop: Segment your test by traffic source as much as possible. Before diving into your test, check out these sources:
- SEO (often mostly branded traffic).
- Emailing and SMS (your existing clients usually outshine the rest).
- Retargeting (these folks already know you; they’re not your average Joe).
- Branded paid search.
Make sure you’re comparing apples to apples in your tests. For example, Google suggests a Performance Max vs. Shopping experiment, claiming it helps you figure out which campaign type rocks for your business. But here’s the catch: Performance Max covers more ad placements than Shopping campaigns. So, the A/B test is a dud from the get-go.
For legit results, compare Performance Max with your entire Google Ads setup, unless you’re using brand exclusions. In that case, pit Performance Max against everything in Google Ads except branded Search and Shopping campaigns.